TLDR: Enterprise AI innovation can fuel your company’s growth and efficiency, but companies face challenges evaluating the range of evolving AI technologies and their opportunities. Here’s how a digital solutions partner can help you identify the highest-impact use cases and manage risk.
Leaders are already seeing how generative AI can dramatically increase organizations’ productivity and growth potential. Engineers are completing tasks in less than half the expected time with the help of tools like GitHub’s Copilot; with ChatGPT, data teams can get code for dataset visualizations in seconds. The use cases for enterprise AI innovation are nearly endless, as teams are beginning to discover.
But that doesn’t mean that the adoption of generative AI technology will be easy or its outcomes guaranteed. To see the transformations possible with this groundbreaking technology, companies should carefully develop an adoption strategy in line with their business requirements, security needs, and opportunities for innovation in their market.
Making the leap with next-generation AI technologies
First, let’s make an important distinction: AI isn’t new. Machine learning is an advanced practice, and many companies have been leveraging this capability for years.
Generative AI and the large language model (LLM) platform launched by OpenAI, however, brings wider accessibility and signals the potential of next-generation enterprise AI technology.
Like predictive AI, generative AI systems are trained on large datasets to provide insights or produce results. But generative AI goes beyond predictive capabilities, creating content based on data in its ecosystem and user prompts. LLM is similarly powerful, using language-based content from its massive training datasets to predict and generate responses, interacting with users via text.
That potential still comes with risks, especially as the technology continues to evolve for commercialization. From employees who query ChatGPT with proprietary company information to prompts that end in obviously false information, enterprises may be eyeing generative AI with a mix of enthusiasm and caution.
Some leaders may be waiting to see the technology mature to the point where a few key pillars are in place — but they’ll need to balance the waiting game with a proactive approach, continually assessing the opportunities and risks of adoption. And eventually, they’ll need to strategically make the leap or risk falling behind innovative competitors actively leveraging enterprise AI’s transformative capabilities.
Pillars of enterprise AI adoption
Enterprises will need to have a high degree of confidence in generative AI — and they’ll need the technical know-how to ensure that AI systems are effectively designed and optimized in a couple of key areas.
- Highest degree of accuracy in business-critical functions. In high-risk industries like financial services and life sciences, the cost of inaccuracy can be serious. Leaders will be thinking about the edge cases where LLM and generative AI fail to generate accurate results, like incorrect market data or inaccurate patient recommendations. But that doesn’t mean companies should dismiss these technologies. Understanding where and how to deploy LLM as the technology evolves is critical — along with establishing the necessary oversight of AI systems to manage implementation, iterate, and fine-tune their performance.
- Privacy protections for company and customer data. In the ChatGPT platform, user input becomes part of the OpenAI data ecosystem — which can prove a complex challenge for businesses to navigate. This model could pose the risk of company or customer data exposure, for instance.
As generative AI systems evolve and companies work with AI experts to implement guardrails, enterprises will be able to limit where sensitive data travels within and outside of the organization.
Leveraging innovation partnerships to power ahead with enterprise AI
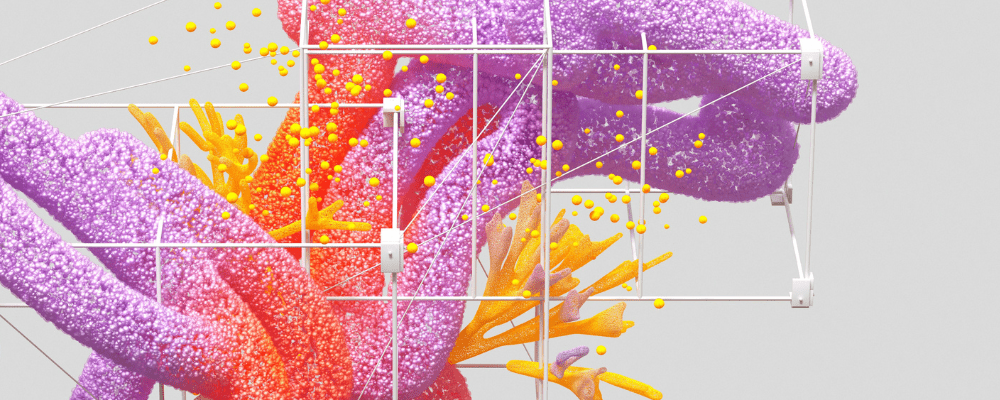
As leaders look to the future of generative AI and LLM for their organizations, they’re coming up against challenges. Whether it’s experimenting with brand new technologies and sorting use cases, the task of transforming with AI is proving a tall order — and one that leaders must respond to as quickly as possible to capitalize on the value potential.
With the constant pace of new development, it may be essential to support your own AI experimentation with an innovation partner, complementing the power of your teams with AI expertise. From rapid proof of concept development to exploring technologies, learn more about how an innovation partner can guide you in implementing next-generation AI.
Evaluating AI technologies & third-party partners
From ChatGPT to the growing range of generative AI-powered productivity tools coming to the market, companies have to sort through all the brand-new AI technology, assessing data privacy features and risk potential as it rapidly evolves and comes online.
While OpenAI and the GPT models are in the headlines now, the AI space is quickly changing and expanding. Other platforms and providers may now or soon be offering solutions better suited to unique business needs.
Along with a supporting innovation team, companies will want to evaluate AI technology features like:
- Data privacy and control. As mentioned, data privacy is a crucial risk factor as companies deploy AI-powered software. Consider that different AI technologies and providers have different data privacy and data control structures. For instance, users add input and data to OpenAI’s LLM — and for now, that means company data could become part of the OpenAI data environment. But other large language model providers allow companies to add the model to their data, granting greater control over data privacy.
- Third-party data sources. Companies may need to leverage third-party data sources as part of their enterprise AI systems, which can add to the complexity involved in finding and vetting technologies.
Identifying the right AI technology solutions for your business requires careful analysis and planning. And it may be an equal risk to delay the process, waiting until new generative AI technology is packaged with enterprise software and comes to market down the line.
Expert digital partners can guide you across all stages of enterprise AI exploration, starting with the technical analysis — and help you start innovating sooner rather than later.
Identifying highest-impact use cases
Waiting too long to explore the potential of generative AI will leave companies at risk of falling behind disruptive competition. But that doesn’t mean that enterprise AI will be a solve for every business case, especially at the outset.
Strategically exploring enterprise AI early on for targeted, high-performing, and high-impact use cases will have transformative potential for organizations. While dozens of use cases are on the table, your innovation partner can help you determine the low-hanging fruit and the highest-impact opportunities for your business.
Here are a few common use cases companies and their enterprise AI innovation partners are likely to look at first.
- Search and knowledge base: When search is paired with generative AI, the user’s experience is transformed from a simple list of results to a comprehensive answer.
- Chatbots, conversational apps, and operational support: By providing support for customers, IT, and engineering, AI can revolutionize the customer experience. AI-powered chatbots will drive a personalized experience, and behind-the-scenes improvements that utilize AI will only improve it.
- Business intelligence and data querying. With generative AI tools, companies can unlock and query crucial data, gaining insights to drive better decision-making.
- Sales insights. AI and generative AI can power sales teams by tracking patterns in lead activity and creating personalized sales collateral.
- Business workflow automation. With AI-powered workflow assistance, teams can automate the most complex tasks seamlessly.
- Cloud cost optimization. Companies requiring intensive data storage or analytics can leverage AI to automate and optimize the performance of the highest-cost cloud functions.
Financial services use cases
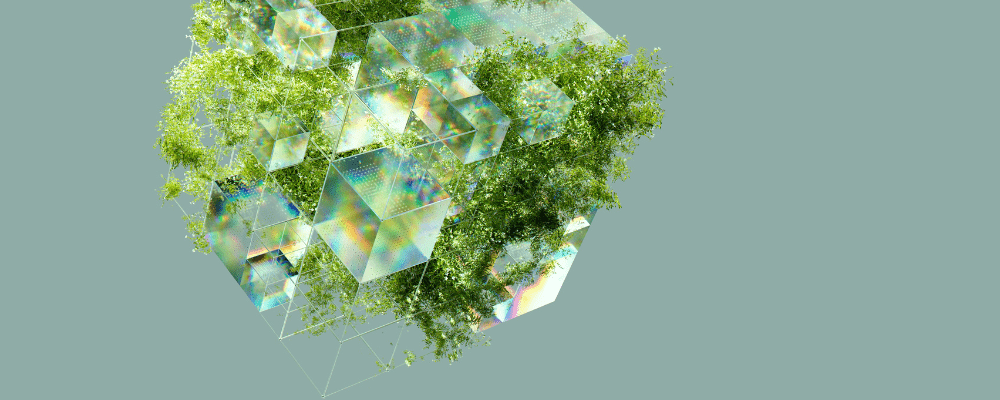
ChatGPT is only the first major signal of what’s possible for generative AI technologies. While GPT is trained on general data from across the web, companies will begin to leverage enterprise AI tech trained on industry-specific datasets, powering even greater potential for use cases and innovation within verticals.
From banking to capital markets to payments, financial services organizations have the potential to build on the growing capabilities of AI by leveraging the next generation of AI advancements.
1. Payments automation & optimization
Companies no longer need to rely on manual analysis for many of their costliest payments challenges. With AI-powered analytics evaluating transaction data or automating operations, card companies can optimize payments processing — from transaction decisioning to chargeback review and prevention.
2. Risk management
Similarly, financial institutions have been leveraging machine learning and AI to manage risk and make better, data-driven decisions. Teams can utilize AI and LLM technologies to automate risk monitoring data.
3. Personalized marketing & customer retention
Customer service is an important factor for brand loyalty among 96% of consumers — and customer service can be transformed and optimized with LLM solutions.1 Companies have significant potential to innovate the customer journey with the latest AI, from cardholder microtargeting to engaging chatbot experiences.
Life sciences use cases
Across life sciences, next-generation enterprise AI will transform everything from clinical trial data and design to the personalization of medicine.
1. Drug discovery
Over 30% of drug discovery will be driven by generative AI technologies by 2025.3 Research shows that the cost of bringing a drug to market ranges from $314 million to $2.8 billion.
2. Synthetic data
As in finserv, patient data privacy is also a significant concern in research and development settings, where exposing medical records can pose risks. Generative can help to produce synthetic data for healthcare and life sciences applications, ensuring minimal concerns around data privacy.
3. Precision medicine
With the power of next-generation AI to learn from large patient datasets, identify trends, and make personalized predictions or recommendations, precision medicine can see significant potential with the latest AI technologies.
Implementing effective design and guardrails
Generative AI can drive game-changing innovation across your organization. But companies will also need help with developing and implementing the tech effectively, including in the following areas.
- Internal search and activity restraints. You don’t want users to run free with just any functionalities of LLM-powered software. Your tech team needs to help you devise guardrails, from the data available to system users to the kinds of activities available through user-based prompts.
- Customer engagement and customer service constraints. Imagine customers asking a GPT-powered chatbot for sensitive data about other customers, for instance. You’ll need rules in place to ensure that AI-powered customer communication is appropriate and limited to certain use cases.
- Data control and data management. It’s crucial to ensure that data testing is in place, especially in testing for risk cases. There will also need to be standards for and management of customer permissions and legally compliant data sourcing, along with constraints on data exposure and sharing capabilities across the enterprise.
Rapidly building & launching prototypes
Ideating and imagining the possibilities of AI is only the beginning. Your in-house innovation team may need a lab of developers who can realize the vision — fast.
AI experts can design, develop, test, and implement custom AI solutions. Whether you need to explore solutions, experiment with design concepts, or rapidly prototype software, a digital partner can help you to quickly scale and deliver innovative enterprise AI technologies.
Build your enterprise AI roadmap with Blankfactor
Exploring and building AI-powered solutions should be done strategically with a technical team that understands your business, security needs, and how to leverage generative AI technologies to produce the results you want.
At Blankfactor, we can help companies lead the way with enterprise AI. Our digital innovation experts have the depth of experience you need to ensure that your data is secure, compliant, and your AI systems drive impact. From financial services to life sciences, our team is ready to engineer the next-generation enterprise AI innovation you need to pull ahead.
Ready to brainstorm your enterprise AI roadmap? Our industry experts and experienced technical consultants can strategize custom solutions tailored to your business in just an hour. Contact our team today for your 60-minute solution session.
Resources
- https://risk.lexisnexis.com/insights-resources/research/us-ca-true-cost-of-fraud-study
- https://www.forbes.com/sites/blakemorgan/2019/09/24/50-stats-that-prove-the-value-of-customer-experience/?sh=120dddad4ef2
- https://www.gartner.com/en/articles/beyond-chatgpt-the-future-of-generative-ai-for-enterprises
- https://pubmed.ncbi.nlm.nih.gov/32125404/